Analyzing Areas of Influence in ArcGIS Online
This tutorial describes one type of analysis of areas of influence that can be performed using ArcGIS Online and Google Sheets.
This example examines potential differences in incidence rates for all cancers in counties surrounding natural-gas-fired power plants in the USA versus counties outside those areas.
Literature Review
Cancer
Cancer is a general name given to a variety of different diseases where the body's cells divide without stopping. In normal life, new cells divide, grow, and die in an orderly process that renews the various parts of the body. However, cancerous cells survive when they should die, and extra cells divide without stopping, forming tumors (NCI 2018a).
Cancer can affect all organs and systems of the body, and types of cancer are usually named after the tissues where the cancers form. Skin cancers (carcinomas) are the most common type of cancer (NCI 2018a).
In 2018, there were around 1.7 million new cancer diagnoses in the United States, and around 600,000 people died from cancer. Almost 40% of all Americans will be diagnosed with cancer at some point in their lives. The overall cancer death rate has declined since the early 1990s, although rates for some cancers have increased (NCI 2018a).
Risk Factors
It is usually not possible to know the exact causes for any specific incidence of cancer. However, there are a variety of risk factors (NCI 2018b) that increase the probability that a person can develop cancer, including:
- Age
- Diet
- Obesity
- Tobacco use
Environmental factors that increase the risk of cancer include:
- Radiation exposure
- Sunlight exposure
- Carcinogens
Study Area: Cancer
The study area for this example is the United States. Although different types of cancer have different spatial distributions throughout the country, overall cancer rates are especially high in the Northeast, Appalachia, and Louisiana.
Cook (2015) notes that despite the patterns for incidence described above, cancer death rates are noticeably higher in the Midsouth and Midwest. Cook attributes differences in incidence and death rates to behavioral differences (like smoking), as well as differences in availability of medical care (early screening), and demographic differences.
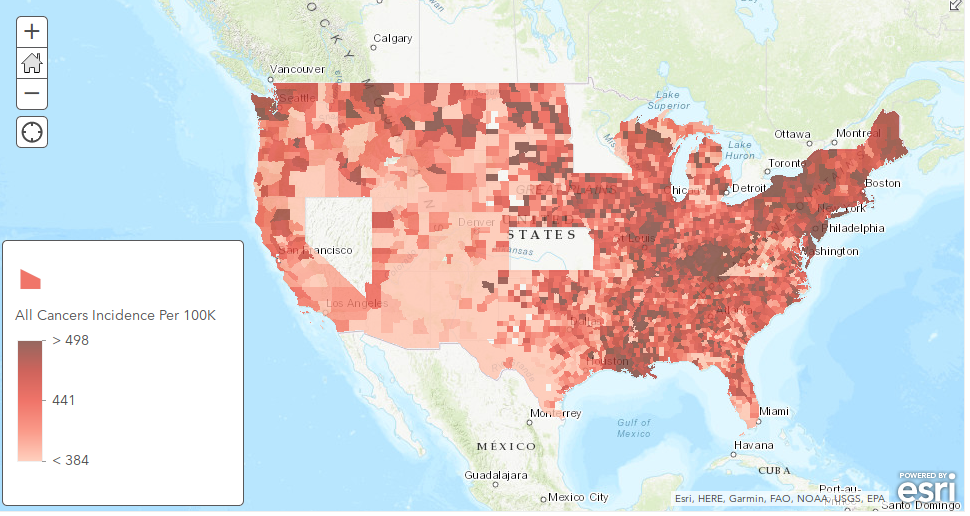
Study Area: Natural-Gas-Fired Power Plants
Natural-gas-fired power plants operate by burning natural gas in a gas turbine, which turns a generator, which generates electricity. Additionally, in advanced combined-cycle plants, the heat from the turbine is used to boil water into steam, which spins a second turbine and generator and more-fully utilizes the energy in the gas and increase plant efficiency. There are around 1,800 natural-gas-fired power plants in the United States representing around 450 gW of generating capacity or around one third of US electrcity use (Afework et al 2018, EIA 2018).
Natural gas emits 50 to 60 percent less CO2 compared to coal-fired plants and produces very limited amounts of the sulfur, mercury, and particulate pollution commonly associated with coal-fired plants. Accordingly, natural gas is considered to be more environmentally-sensitive than coal, and switching from natural gas to coal is associated with public health benefits (UCS 2018).
While there appears to be little literature on cancer risks associated with the burning of natural gas, the increased production of natural gas to fuel the move away from coal is associated with increased air pollution, and those pollutants are linked to cancer (UCS 2018, Vogel 2017).
References
Afework, Bethel, Jordan Hanania, James Jenden, Kailyn Stenhouse, and Jason Donev. 2018. "Energy education: Natural gas power plant." Accessed 26 October 2018. https://energyeducation.ca/encyclopedia/Natural_gas_power_plant
Centers for Disease Control and Prevention and The National Cancer Institute (CDC). 2018. "State Cancer Profiles, Incidence Rate Report for United States by County, 2010-2014." Accessed 15 May 2018. https://www.statecancerprofiles.cancer.gov/incidencerates/.
Cook, Lindsey. 2015. "You're Most Likely to Die From Cancer in 1 of These States." US News and World Report, 15 October. Accessed 27 October 2018. https://www.usnews.com/news/blogs/data-mine/2015/10/15/youre-most-likely-to-die-from-cancer-in-1-of-these-states.
National Cancer Institute (NCI). 2018a. "Cancer Statistics." Accessed 12 October 2018. https://www.cancer.gov/about-cancer/understanding/statistics.
National Cancer Institute (NCI). 2018b. "Risk Factors for Cancer." Accessed 12 October 2018. https://www.cancer.gov/about-cancer/causes-prevention/risk.
Union of Concerned Scientists (UCS). 2017. "Coal and Air Pollution." Accessed 16 November 2018. https://www.ucsusa.org/clean-energy/coal-and-other-fossil-fuels/coal-air-pollution.
Union of Concerned Scientists (UCS). 2018. "Environmental impacts of natural gas." Accessed 26 October 2018. https://www.ucsusa.org/clean-energy/coal-and-other-fossil-fuels/environmental-impacts-of-natural-gas.
US Energy Information Administration (EIA). 2018. "U.S. Power Plants." Accessed May 15, 2018. https://www.eia.gov/maps/layer_info-m.php.
Vogel, Lauren. 2017. "Fracking tied to cancer-causing chemicals." Canadian Medical Association Journal 189 (2): E94 - E95. Accessed 26 October 2018. https://www.ncbi.nlm.nih.gov/pmc/articles/PMC5235941/.
Question and Hypothesis
Our research question is, "Do counties surrounding natural-gas-fired power plants have higher rates of cancer than areas outside those areas of influence?"
Although there is research linking coal-fired plants to cancer (UCS 2017), there does not appear to be a significant body of research linking proximity to natural-gas-fired power plants and cancer.
Therefore, our hypothesis is, "Counties within 25 miles of natural-gas-fired power plants do not have significant differences in overall cancer rates compared to the rest of the USA."
Methods and Data Sources
Counties within 25 miles of specific energy plants were considered potential areas of influence. The means of cancer rates and survey margins of error were compared between areas of influence and all other US counties.
Our cancer data source is the "State Cancer Profiles, Incidence Rate Report for United States by County, 2010-2014," from the Centers for Disease Control and Prevention and The National Cancer Institute. The data may be accessed here.
Our power plant data for 2018 is from the US Energy Information Administration. The data may be accessed here.
Map the Areas
The first step in analyzing the geospatial distribution of a phenomena is generally to map it and look for patterns.
The video below demonstrates the creation of a choropleth using a cancer rate feature layer already created in this ArcGIS Online organization. In this case we symbolize by the incidence rate (per 100,000 population) for all cancers.
Although there are hot spots (clusters) of cancer around the country, there is a notable cancer belt that extends from the deep south through Appalachia.
Map the Points
Areas of influence are centered around specific points. The video below demonstrates adding points from a power plant feature layer already created in this ArcGIS Online organization.
Filter Points
In some cases you may need to filter your points to isolate a specific type of influence. For example, this feature layer contains points for all types of power plants. The video below demonstrates how to filter the layer so that only the plants with natural gas as their primary fuel source are displayed.
We also color the points black so they will stand out over the completed map.
Find Nearest Locations
To isolate the counties around the power plants that we hypothesize may have higher cancer rates, we use the Find Locations, Find Existing Locations analysis tool.
- For this example, we ADD EXPRESSION to select counties that have at least some area within a distance of 25 miles (arbitrarily chosen) from a plant
- Give the resulting layer a meaningful name. You may want to include your last name in the name to avoid duplicating a name used by someone else in your organization
- Uhcheck Use current map extent so that any areas outside the area currently being mapped will be selected
- Show credits to make sure you haven't made a mistake that will use up your credit quota. Credits required over 10 generally indicate some problem
- We symbolize them by cancer rate with a red color ramp so the will stand out in the map
- Create a new Google Sheets spreadsheet from Google Drive
- Give the spreadsheet a meaningful name
- Type in the names for the bars, the cancer rates, and the margins of error
- Select just the first bar name and cancer rate
- Insert -> Chart and make sure it is a Column chart
- Switch rows / columns so column A becomes the name of the series in the legend
- Add Series and select the second bar name and cancer rate
- Customize -> Series and turn on Error bars
- Apply to: the first series
- Change the Type to Constant and enter the margin of error for the first bar
- Repeat for the second bar
- Add a meaningful Y axis title
- Adjust the vertical axis to show the full range of the bar
- Move the chart to its own sheet and publish the chart to get a shared link or an iframe
- Areas of influence can be based on analysis of air and water flows rather than arbitrary 25-mile areas of influence
- The research could focus on where diagnosed individuals have lived and worked to investigate the exposure to environmental carcinogens in the past
- Spatial lag regression is variation on simple regression that takes into account spatial autocorrelation
To isolate counties outside that area, we repeat the operation, but choose not within a distance of. We symbolize them blue so they are visually subdued compared to the red areas of influence.
Share the Map and Layers
Results
Statistics
Now that we have a layer of areas that should be influenced by the points, and an area that should not be influenced, we can compare the statistics to see if there is any difference in the dependent variable - in this case incidence rates for all cancers.
The average cancer rate for all counties within 25 miles of a natural-gas-fired power plant is 443.98 new annual diagnoses per 100,000 population. The average cancer rate for counties outside 25 miles of a natural-gas-fired power plant is lower at 430.05. This indicates that the cancer rate is slightly higher near gas power plants.
However, because the cancer data is sampled data, it has a margin of error. The average margin of error for gas counties is 32.08, so the 95% confidence interval for the cancer rate is between (443.98 - 32.08) and (443.98 + 32.08) or in the range of 411.9 to 476.06. The average margin of error for non-gas counties is 54.2, meaning the 95% confidence interval for cancer rates in non-gas counties is 375.85 to 484.25.
Because our confidence intervals overlap and we cannot be certain that the cancer rate in counties over 25 miles from a natural-gas-fired power plant is actually higher than the rate inside 25 miles, we have corroborated our hypothesis that counties within 25 miles of natural-gas-fired power plants will not have significant differences in overall cancer rates than the rest of the USA.
Visualization
We can visualize our two cancer rates and the overlap between the confidence intervals by using a bar chart with error bars that show the confidence interval around the mean. Note that the range of confidence interval values on the vertical axis encompassed by the bars overlaps, indicating that we cannot be certain there is any difference between the two bar values.
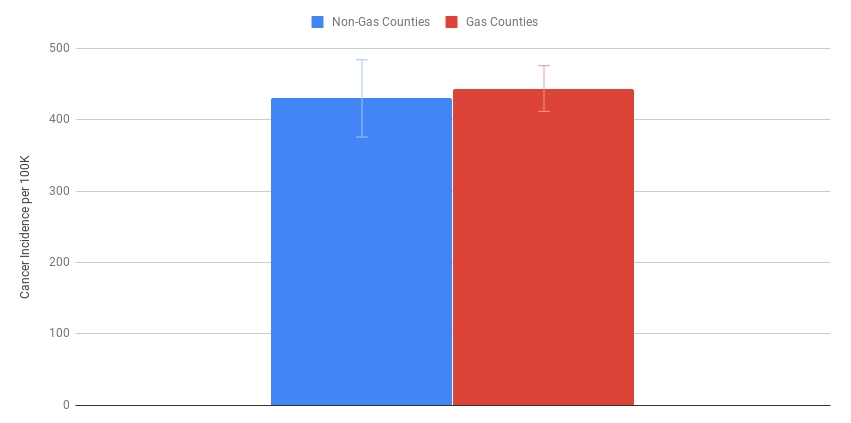
Empirical Limitations: Problems With Data
While this comparatively simple type of analysis gives intuitive results with a minimum amount of effort, it is subject to a number of limitations that should be noted when attempting to interpret the results of the analysis.
Data Quality Problems
Survey data subject to a number of issues that affect its accuracy. For example, areas with sparse populations limited medical facilities might underreport cancer diagnoses to researchers. People in some areas might also be suspicious of or unresposive to researchers, resulting in less-accurate data.
Missing data can also be a problem. In the example above, no county-level data is available from Nevada, Minnesota, and Kansas, and data for some specific cancers is unavailable for a number of rural counties. This biases results in favor of more-populous counties.
Spatial Lag
Point-source pollutants are often spread by wind and water currents far beyond the source. This can both reduce the effects of those emissions (dilution is the solution to pollution) or concentrate those pollutants downwind or downstream. Pollution can become concentrated in rivers as those rivers flow past multiple sources of pollution.
Human mobility is also a problem. Because people usually change locations during the day (home, work, school) and occasionally relocate residences, the effects of carcinogen exposure may not become visible in the area where the actual exposure took place.
This is a variation on the modifiable areal unit problem (MAUP), where changing the areas used for analysis can change the results of the analysis, even if the underlying phenomena is the same.
There are numerous ways of minimizing (but not eliminating) issues with spatial lag:
Temporal Lag
Cancer often takes months or years to develop. Accordingly, there may be a temporal (time) lag between exposure(s) and diagnoses of cancer. Many people may also move between the period of the exposure and the onset of disease. Such temporal lag can hide clear connections between carcinogens and cancer.
With long-lived assets like power plants that persist in communities for decades, this effect is reduced. However, when studying the effects of new facilities, observations over extended periods of time (longitudinal studies) and statistical techniques that take temporal lag into account are needed to compensate for temporal lag. Indeed, temporal lag can become a variable in the analysis when increased cancer rates follow the opening of a new plant.
Qualitative Aggregation Problem
Using data that aggregates (combines) different diseases or groups into a single set of values can hide important differences.
For example, power plant emissions might be expected to have a greater impact on certain organs, such as lungs or breasts. Since the analysis in this example used a cancer rate that included all different types of cancer, increased rates for specific types of cancer might get diluted in the aggregated value.
This issue can be mitigated by focusing on specific types of cancer.
The Small Numbers Problem
In areas with small populations, the occurance (or absence) of one rare health condition can have an outsized influence on the incidence or prevalance rate for that area. Because there are a large number of counties with small populations in the US, these extremes can distort the descriptive statistics derived from those values.
Rational Limitations: Problems Of Interpretation
While correlation may be interesting, what we are usually more interested in is causation - whether one of the phenomena we are observing causes the other phenomena. In this example, we are interested in whether being close to a natural-gas-fired power plant causes cancer. Such knowledge could be used to justify tighter emissions regulations for power plants, or to help promote public funding to accelerate alternative energy research and deployment.
While correlation is empirical (based on observation), causation is based on reason. When we observe two phenomena occurring together and we observe that there is some mechanism, we use reason and logic to tie those two phenomena together in a cause and effect relationship.
Assuming that correlation indicates causation is the post hoc fallacy, from the Latin phrase post hoc ergo propter hoc (after this, therefore because of this). A logical fallacy is an often plausible argument using false or invalid inference.
In this example, if we had found a higher rate of cancer near natural-gas-fired power plants, we could have inferred a causal relationship between proximity to power plants and cancer. Likewise, since we found no difference in cancer rates, we infer that there is no relationship between natural-gas-fired power plants and cancer.
If the only evidence we have for this inference is this analysis of correlation between proximity to power plants and cancer, we fall into the post hoc fallacy for reasons explained below.
Covariates
Covariates are additional independent variables that might influence the dependent variable.
As described in the literature review, cancer has numerous risk factors. In this example, we only looked at one of those variables (proximity to power plants). A more sophisticated analysis would consider covariates in an attempt to determine how important each one of those variables are in explaining different county cancer rates. Common analytical techniques used to do this include multiple linear regression analysis, principal component analysis, and factor analysis.
For example, a regression model might consider not only whether a county is near a natural-gas-fired power plant, but also incorporate covariates that quantify lifestyle risk factors like obesity rates, rates of regular consumption of fruits and vegetable, and rates of smoking. The resulting model would contain coefficients that indicate the relative percentage of influence on cancer rates that can be attributed to each of the variables.
Confounders
A confounder is a third variable that correlates with both the presumed cause and effect variables. If we had found a correlation between proximity to power plants and cancer, this issue may have been present.
For example, power plants are often located in poor areas where residents have little political power. Because lifestyle and medical care issues associated with poverty could also increase cancer rates, those factors could increase cancer rates around power plants while the emissions from the power plant actually have no carcinogenic effect.
Ecological Fallacy
The ecological fallacy is the use of aggregated statistics to explain individual situations.
For this example, the causes of cancer are complex and often specific to individuals (such as genetics or lifestyle). If someone near a natural-gas-fired power plant gets cancer, using this aggregated data to make an argument that the power plant had nothing to do with their cancer would fall into the ecological fallacy.
Similarly, a small group of dirtier power plants might be emitting carcinogens that affect people in their vicinity, but since those numbers are aggregated with all other power plants, the effects of those specific plants would not show up in the aggregated mean. Using this analysis to argue that no natural-gas-fired power plants increase the rate of cancer in their vicinity would also fall into the ecological fallacy.
Biography
Taylor Swift is a Science, Technology, and Society major in the Department of Science, Technology, and Society at Farmingdale State College, with a concentration in Health, Wellness, and Society. She currently lives in New York City. Her research interests include energy, futures, and open-source software. Her career plans include continuing to be an internationally-renown pop music icon.